Projektteam
J. Sasse, R. Jenke, RWU Ravensburg-Weingarten University ofApplied Science
L. Prasolb, Hilti AG
1.Problem statement
- High costsformillingtoolsin machining (Fig. 1) and missingroot cause:
- Notransparencyaboutreal toolwear
- Different operatinglifetimesdue toheuristics-basedsetupandexchangeofmillingtoolsbyworkers
-
Task: Perform association analysis to determine relationships and influencing factors on tools‘ lifetime* and determin suitable measures to optimize tool usage in machining
(*measured by parts produced during lifetime)
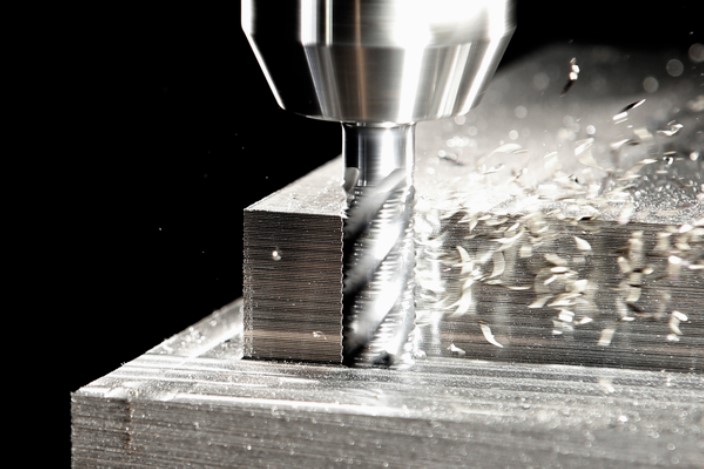
2.Data processing
3.Method
4.Results
5.Future Perspective
- Individual tool settings for each tool-part-combination to optimize tool usage
- Use of model as data-based decision support regarding tool exchanges and tool life adjustments which could also be replenished by cost/time optimized tool changes (Fig. 3)
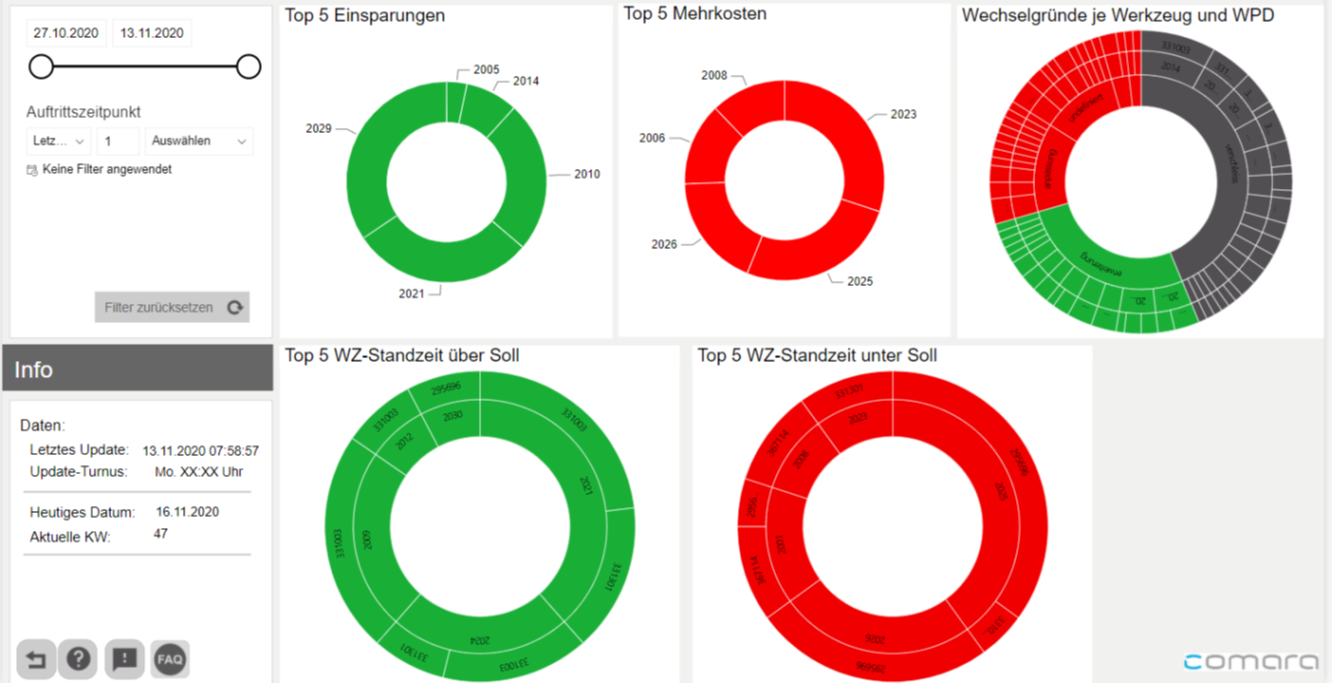
Literature
- Sasse, J. (2020): Process Mining in der Produktion –Durchführung einer Datenanalyse in der spanenden Fertigung. Master Thesis, RWU.
- Cleve, J. & Lämmel, U. (2016): Data Mining. De Gruyter Studium, 2nded., Berlin: De Gruyter.
- Kröckel, J. (2019). Data Analytics in Produktion und Logistik. 1. Auflage, Vogel